AI has made remarkable progress in almost all its sub-areas except chaos. Chaos theory deals with unpredictable time evolution of many nonlinear & complex linear systems as best illustrated by Lorentz’ famous butterfly effect. In 2007 Edward Lorentz, the father of chaos theory concluded, “long-term climate forecasting is impossible.” Today, Lorenz would be astounded by the progress machine learning (ML) has made to counter his predictions. Weather is a level 1 chaos that does not react to predictions. Although it is influenced by myriad factors, we can now model ML to produce better weather forecasts. However, Level 2 chaos that involves human reaction to prediction that changes its outcome, can never be predicted accurately. Equity markets, for example, are a level 2 chaos that even the best ML model cannot predict.
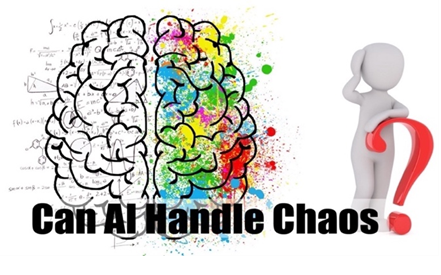
In fact, almost every ecosystem involving human decision making is level 2 chaos, because knowledge tends to affect people behavior making level 2 chaotic systems impossible to predict even if AI reaches singularity (machine intelligence exceeding human intelligence). Collective Artificial Super Intelligence (CASI) is a novel ML approach that breaks the singularity barrier. In contrast to traditional AI systems, collation of intelligence of individuals is not a zero-sum game; it is multiplicative, and coming from collective wisdom of many it can potentially reach superhuman levels. We develop, test & validate feasibility of CASI in 3 challenging human empowering use cases that introduce predictability, objectivity, transparency & accountability into computer-human interaction providing for a truly mixed human-AI autonomy for improving democratic governance of human-machine initiatives. A multidisciplinary consortium designs CASI as an approach that puts humans at the center, changing the course of the AI revolution to achieve super-intelligence even before singularity is reached. CASI targets those unassailable aspects of human-machine interactions.